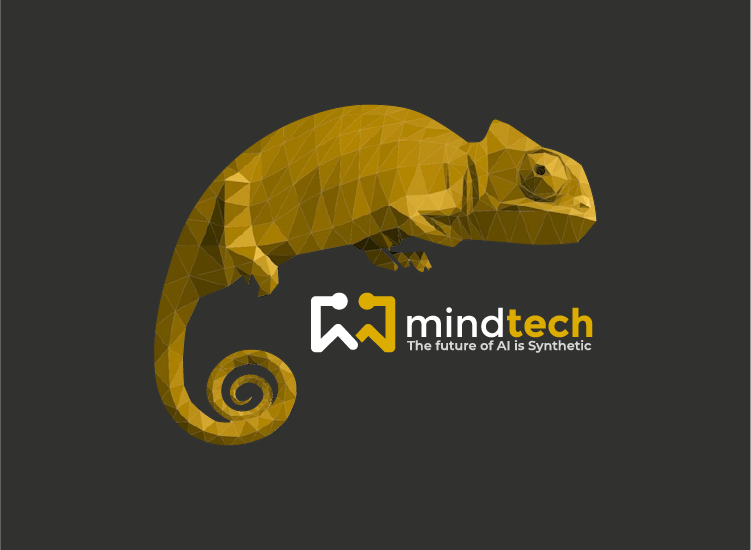
Share article:
Tags:
Mindtech on Synthetic Data: What You Need to Know
To train an AI vision system to see, understand, and respond to what happens in the real world, you need to provide it with a certain amount of data (annotated images) related to the real-world scenarios it’ll be deployed in. Depending on the application, and required accuracy, it’s estimated that 100,000s or 1,000,000s of high-quality images are required to generate an adequate amount of training data.
However, privacy laws, and restricted access to scarce data sets – not to mention the time-consuming job of data labelling – have in the past been obstacles for developers in training AI vision systems. Synthetic data is a solution to help companies overcome these issues.
What is synthetic data?
Synthetic data, in the context of AI vision systems, is annotated imagery that has been taken from computer-generated, photo-realistic, 3D environments. These environments contain items, vehicles, people – anything that exists in the real world – that are placed in scenarios ranging from the everyday to the extreme. For example, an engineer training a home security vision system would run scenarios featuring a delivery person and a would-be intruder so it can learn when to sound the alarm. The annotated images and metadata created are then used to train AI vision systems.
Our Chameleon platform allows users to create their own scenarios like these, or select from pre-set ones. Check out the platform in our video here.
How does it work with real-world data?
Synthetic data compliments real-world data. Research from Cornell University suggests good training results come from data sets with 90% of synthetic data and 10% of real-world data, though this balance varies depending on the application.
What are the benefits of this approach?
Synthetic data allows companies training AI vision systems to be more efficient and privacy-compliant, and makes scaling up more straightforward. This is because they can use synthetic data software creation platforms to generate the bulk of the images needed in a couple of days, instead of months.
And because the data is computer-generated, there are no privacy concerns, while biases that exist in real-world visual data can be addressed too. In the virtual world, different ethnicities, age groups, and diversity in terms of colour of clothing or sex are much easier to create. And as data changes over time, it’s easier to reflect this in a virtual environment to avoid data drift impacting an AI model’s performance.
Synthetic data for corner cases or scenarios (camera location modelling, different lighting, and other variables), which would be hard to create in the real world, can be quickly and easily created in a 3D virtual environment. Extreme, ‘nightmare’ scenarios—a catastrophe or a crime, for example—can be also simulated risk-free to create the kind of data that’s difficult to come by from real-world sources.
Synthetic data helps solve a bottleneck in AI training, and we’re already seeing its value in multiple cases – for example, in healthcare to train machines to monitor patients recovering from surgery, and in security and safety systems to detect suspicious objects or unusual patterns of behaviour. Now, a ‘best of both worlds’ approach to training, combining real-world and synthetic data, will result in even smarter, safer systems.