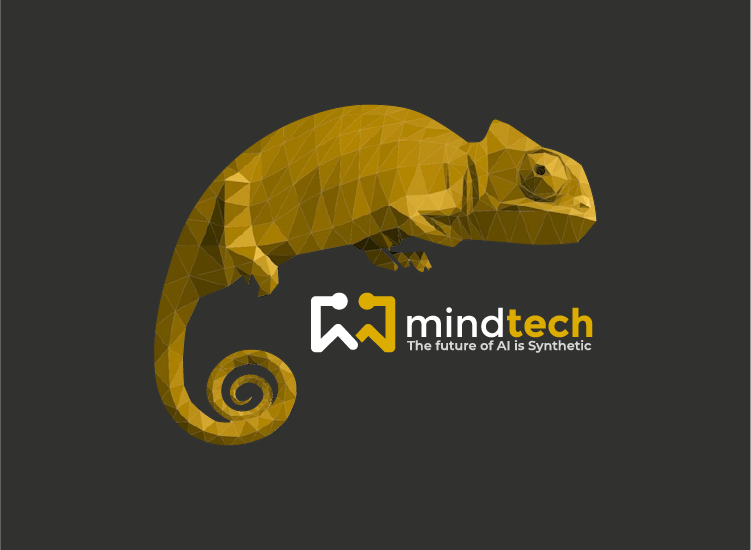
Share article:
Tags:
Mindtech has technology that can further improve road safety by leveraging synthetic data to enhance the accuracy of text and sign detection systems. By focusing on reducing false positives, tackling challenging scenarios, and addressing unique cases where real-world data may be scarce, Mindtech’s cutting-edge approach uses both fully synthetic, as well as hybrid (real/synthetic mix) data to create key training data .
False positives in text detection can lead to erroneous actions, miscommunications, and even security concerns. Mindtech has taken a forward-thinking approach to combat this issue by harnessing the power of synthetic data, and in using multiple techniques such as domain matching, the use of context and invariance images, as well as hybrid data, curated using our data analysis platform Dolphin, blending real-world text data with carefully crafted synthetic samples, our models achieve a level of precision that minimises false positives significantly. End users understand that accuracy is the key to a frustration-free experience, with even fractions of a % improvement making a significant difference to user experience.
Text detection systems often encounter challenging scenarios that can impede accurate results. Factors like low-light conditions, partially obscured text, or complex backgrounds can pose significant obstacles. However, Mindtech’s innovative synthetic data library simulates these diverse challenges, exposing their algorithms to a myriad of scenarios they may face in the real world. As a result, Mindtech-equipped text detection systems can triumph over these difficulties, unlocking new possibilities in document digitisation, security, and accessibility.
Case study: traffic signs
By way of example, Mindtech recently conducted a self-initiated project with a focus on detecting text on traffic signs. We used an existing pre-trained text detection network that worked with text in generic scenes, but also underperformed when it came to identifying text on traffic signs. The problem needed to be solved as quickly as possible using an efficient method.
The solution? We were able to obtain and label real-world test images that represent typical cases the system will be expected to identify. By identifying the sub-domain of interest for this particular text detection task, we could then develop a framework for generating synthetic data. Creating synthetic images, we could augment the dataset to cover the failure cases and complement the original dataset. Essentially, we were able to re-train the network using the synthetic images and check against the test dataset.
In certain applications, text detection encounters unique cases where real-world data is scarce or non-existent. This could include identifying specific road signs, symbols, or languages that are rarely encountered. Mindtech’s synthetic data capabilities shine in these situations, enabling the creation of lifelike representations of rare text samples. By doing so, Mindtech empowers text detection systems to comprehend and respond to these exceptional cases.
As we look ahead, the synergy of synthetic data and text detection promises a multitude of opportunities for societal advancement. To find out more about how Mindtech’s synthetic data solutions can benefit you, reach out here.
Text Detection for Good: Empowering Society with Synthetic Data
In the realm of computer vision, text detection plays a vital role in a wide array of applications, from document analysis to smart city initiatives. For example, Mobileye recently made headlines for launching the first camera-only Intelligent Speed Assist system to comply with new EU standards. The system utilises advanced camera technology to assist drivers in identifying different information, including speed limits, all to improve road safety.
Mindtech has technology that can further improve road safety by leveraging synthetic data to enhance the accuracy of text and sign detection systems. By focusing on reducing false positives, tackling challenging scenarios, and addressing unique cases where real-world data may be scarce, Mindtech’s cutting-edge approach uses both fully synthetic, as well as hybrid (real/synthetic mix) data to create key training data .
False positives in text detection can lead to erroneous actions, miscommunications, and even security concerns. Mindtech has taken a forward-thinking approach to combat this issue by harnessing the power of synthetic data, and in using multiple techniques such as domain matching, the use of context and invariance images, as well as hybrid data, curated using our data analysis platform Dolphin, blending real-world text data with carefully crafted synthetic samples, our models achieve a level of precision that minimises false positives significantly. End users understand that accuracy is the key to a frustration-free experience, with even fractions of a % improvement making a significant difference to user experience.
Text detection systems often encounter challenging scenarios that can impede accurate results. Factors like low-light conditions, partially obscured text, or complex backgrounds can pose significant obstacles. However, Mindtech’s innovative synthetic data library simulates these diverse challenges, exposing their algorithms to a myriad of scenarios they may face in the real world. As a result, Mindtech-equipped text detection systems can triumph over these difficulties, unlocking new possibilities in document digitisation, security, and accessibility.
Case study: traffic signs
By way of example, Mindtech recently conducted a self-initiated project with a focus on detecting text on traffic signs. We used an existing pre-trained text detection network that worked with text in generic scenes, but also underperformed when it came to identifying text on traffic signs. The problem needed to be solved as quickly as possible using an efficient method.
The solution? We were able to obtain and label real-world test images that represent typical cases the system will be expected to identify. By identifying the sub-domain of interest for this particular text detection task, we could then develop a framework for generating synthetic data. Creating synthetic images, we could augment the dataset to cover the failure cases and complement the original dataset. Essentially, we were able to re-train the network using the synthetic images and check against the test dataset.
In certain applications, text detection encounters unique cases where real-world data is scarce or non-existent. This could include identifying specific road signs, symbols, or languages that are rarely encountered. Mindtech’s synthetic data capabilities shine in these situations, enabling the creation of lifelike representations of rare text samples. By doing so, Mindtech empowers text detection systems to comprehend and respond to these exceptional cases.
As we look ahead, the synergy of synthetic data and text detection promises a multitude of opportunities for societal advancement. To find out more about how Mindtech’s synthetic data solutions can benefit you, reach out here.
Text Detection for Good: Empowering Society with Synthetic Data was originally published in MindtechGlobal on Medium, where people are continuing the conversation by highlighting and responding to this story.