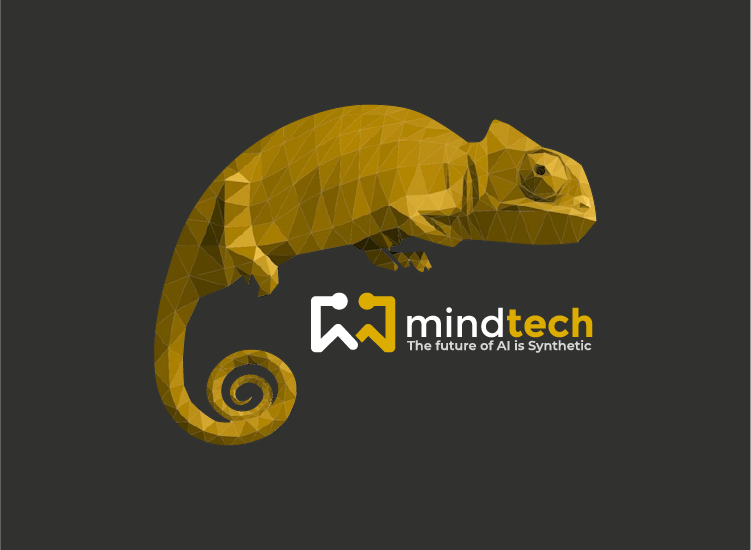
Share article:
Tags:
The use of copyrighted material to train generative AI models like ChatGPT has come under increasing scrutiny over the last year. This has resulted in lawsuits by companies such as Getty Images and the New York Times against AI firms over such practices, which are sure to be talking points this year. In response, Open AI has stated it can’t train its models without such content.
Further challenges also emerge when collecting real-world data in situations such as filming in public spaces, necessitating model releases and approvals. Moreover, government regulations and legislative processes like the EU AI Act further complicate real-world data collection. Navigating the ethical landscape can be subjective and does not have a clear rule book. Ultimately, the decision to regulate AI will be with governments and lawmakers.
Overcoming the privacy challenge with synthetic data
With these legal battles and ethical considerations affecting the availability of real-world data, the industry is facing a need for alternative ways of using data to train AI models. For the coming year, we can therefore expect a steep increase in interest in synthetic images and training data.
Synthetic data offers a solution by being inherently privacy-compliant, enabling rapid and cost-effective data generation. Additionally, it plays a crucial role in testing models, especially for tasks like ID verification, where it allows testing against false information. Industries, particularly those relying on foundation models like ChatGPT models, will benefit significantly from its use.
As synthetic data replaces the need for massive amounts of real data while maintaining privacy, the opportunities for its use are widespread.
Can you tell the difference?
Convincing organisations and governments of synthetic data’s validity, however, remains somewhat difficult, requiring an explanation of what exactly it can do and do well. The challenge lies in persuading stakeholders to embrace new approaches instead of sticking to the status quo. Some businesses may have used older versions of synthetic data and do not know what its new capabilities are.
The last 18 months have seen rapid advancements in the state of the art of Synthetic data usage in the DataOps cycle. Visual fidelity has been pushed through advances in the processing power of the GPUs used for rendering, including wider adoption of techniques such as ray-tracing by the underlying software’ made feasible by the GPU hardware accelerators. Key in closing the domain gap has been the ability of expert companies, such as Mindtech Global, to accurately model the real-world conditions and produce synthetic data, that accurately matches real-world noise, distortions and colour gamuts. New techniques are being used by such companies to verify the network fit of synthetic data with real-world data, to ensure training gives improved results.
A way forward
It’s worth stressing that humans have a vital part to play in overseeing the creation and validation of synthetic data. Data analysts can ensure that it accurately reflects the real world, can adjust datasets to mitigate for bias and accuracy, and can assess any privacy implications that may be in play.
Real-world data is also still a vital part of the process in building synthetic data and training AI models. However, synthetic data can massively reduce reliance on its use and remove any exposure of personally identifiable information and copyright issues. It can even more accurately reflect the real world than using real-world data alone.
Synthetic data provides a way forward for tackling the industry’s tall task of finding privacy-compliant solutions for the new world of AI.
Learn more about why to choose synthetic data here.