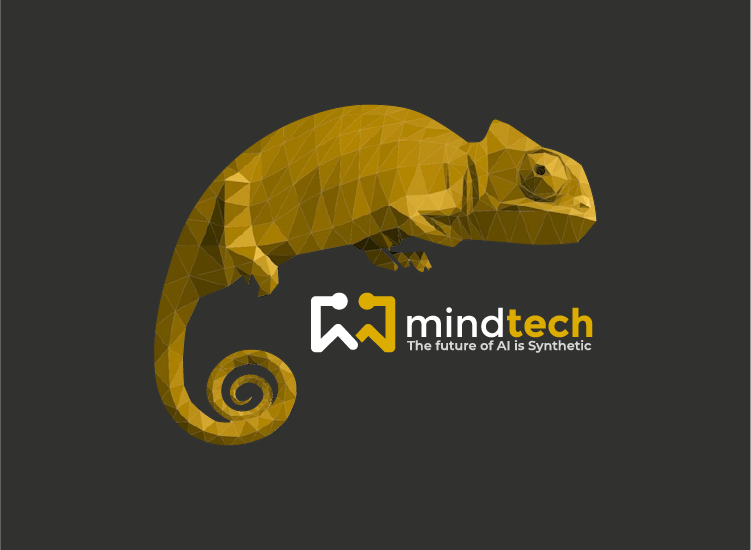
Share article:
Tags:
By Chris Longstaff, VP Product Management, Mindtech
There is no getting away from the fact that to build AI based vision systems that understand the world about them requires massive quantities of labelled images/image sequences.
Therein lies a difficult problem: annotating real-world images is a time-consuming, expensive and frequently inaccurate process often performed by thousands of low-skilled or un-skilled internet crowd-workers. The accuracy can be impaired by the software they use or the tedium of the task. There is even a risk of malicious labelling (as we’ll see later).
Annotating a real world image for a security robot application. Note the overlapping and ill defined humans in the distance, this is a significant problem when annotating.
The task is time-consuming and expensive since, despite the advent of automated software tools that help seed (and so speed) the labelling process, a human still has to oversee the process, and discriminate between difficult key objects, such as adult and child pedestrians (Is it a child closer to the camera, or an adult further away — both have similar heights in the image). What is occluded by a fence? Is the blue, sea or sky? Where exactly should the bounding box go for distant, and occluded/overlapping objects? It’s a problem that is getting worse, as ML engineers and system designers start to use increasing sophistications of annotations, such as instance segmentation, activity labelling, full 3d-pose information.
It all adds up to headaches for machine vision developers — with one of the biggest issues for them being the time it takes to get an image set annotated. An AI model for vision may need of the order of 100,000 labelled frames. As it can take up to an hour to fully annotate a complex image with semantic segmentation, the job could take a data labelling service 16 weeks or so to label all the frames. And checking them will tie up a significant proportion of an ML engineer’s time, too.
That’s far too long a lead time in the fast-moving machine vision space, where reducing time-to-market is vital. And it’s a severe drain on the ML engineering resource, especially for resource constrained start-ups.
That’s why at Mindtech we enable visual AI developers to vastly reduce the number of real-world training images they use by instead utilizing synthetic data: computer-generated images, with pixel-perfect advanced annotation, to train their machine learning networks. In a synthetic imaging platform like Mindtech’s Chameleon, we’ve created the 3D virtual world, we know exactly what objects are in it, and we know where they are in three dimensions — so the image can be annotated automatically, 100% accurately and instantly. On top of that, the synthetic images are, of course, privacy compliant, too.
100% accurate, and advanced annotations available from Mindtech’s Chameleon Synthetic Data Platform
Some real world images are always needed in the process. As an absolute minimum for testing; but usually training gives best results where a few real-world images are mixed with synthetic.
So for those few real-world images, what are the issues with manual annotation that ML engineers and data scientists checking on the labelling quality should be aware of?
First, there are trade-offs to be made between the accuracy of the visual AI application and the number of element types labelled in a real-world image. Fully partitioning all parts of the image into different categories — a process known as segmentation — will mean each frame takes around an hour to label. So to speed things up, fewer element types could be annotated, or a simpler annotation such as bounding boxes can be used, perhaps labelling “human” or “obstruction” only in the example image with the security robot.
Restricting labeling to only 2 classes for reduced time to market and cost. However, this limits the possible functionality and accuracy of the system.
Annotating real world images may be subject to significant errors such as through the inaccuracy of the 2D bounding boxes for example, where the crowd worker’s annotation software automatically snaps around image elements it thinks are correct, and yet are incorrect and go unchecked: the automatic snapping/overlay of these boxes is prone to error — sometimes they only partially cover an object, say, or occlude something important behind it (like a child or a bike) or are simply too big and so cover multiple objects at once. The problem is amplified where segmentation is required.
So it’s vital that images are checked after annotation — a joyless, tedious process that, unfortunately, can take as long as the labelling. And that can be disaffecting for a highly-qualified ML workforce: so again, keeping the number of real-world images used in your AI training as low as possible, will give ML engineers more time to work on algorithms, less time on cleaning data.
Beyond lead time, expense and inaccuracy issues, however, there are two more risks with real-world image annotation that should be considered: the first risk is that of intellectual property theft after sending out your training images to a third party.
Remember, these are the images at the heart of your AI — so if they are leaked by disgruntled crowd-workers, say, they could give clues to your model’s strengths and weaknesses to competitors. At this point, a company has lost control of data that could be central to its competitive advantage — and it opens them up to a raft of attacks.
Theft of that data could allow a rival to build its own model, or work out where there are training gaps in the ML network — for instance, that when built into a security system, the AI cannot see people wearing red clothes, perhaps. This may seem far-fetched, but it’s worth bearing in mind that criminal networks can be just as innovative as the companies they’re looking to target.
The second risk is that outsourcing annotation to the crowd also leaves firms open to potential malicious labelling by bored, badly-paid crowd workers. Simply for fun, or financial gain, they may mis-label handguns as an aerosol can, for example, damaging a security application if the mislabelling is not caught pre-rollout, or labelling a 20 mph sign as 80 mph. Clearly, working with a reputable and proven data annotation company is a must when using real-world training data.
All these issues and risks with labelling shout one thing loud and clear: companies wanting to prosper in visual AI should look at methods to reduce their use of real-world images — and instead, look to boost their use of automatically-annotated synthetic images.
Accelerating Time to Market: How Synthetic Data can alleviate the waiting time for annotated data was originally published in MindtechGlobal on Medium, where people are continuing the conversation by highlighting and responding to this story.