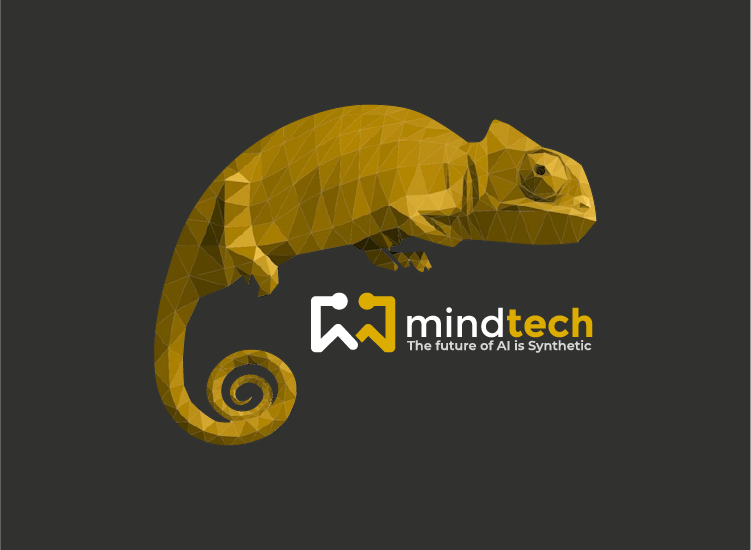
Share article:
Tags:
By Chris Longstaff, VP Product Management, Mindtech
Whether you’re trying to maximize the accuracy of a visual AI model you’re building from scratch, or looking to improve the accuracy of one you’re already working with, mixing synthetic data with real world training data is a sure-fire way to boost a network’s precision.
A “Real” Image Of a quarry area
But how do you decide how many synthetic images to blend in with real ones to optimise your AI model’s performance? There is no simple answer to that question — as there is no magical proportion of synthetic-to-real data that will work across every kind of AI application.
Synthetic Data of workers in PPE in Quarry
Instead, AI developers and data scientists of AI machine vision systems should ask themselves these questions:
· Precisely what is the visual AI model trying to achieve?
· Which uncaptured images could cause the model to fail?
· What mix of synthetic and real images will ensure success?
As an example, imagine a machine vision model that’s designed to sound an alarm when people stray too near a railway line. In bright sunlight, it’s 100% accurate at detecting people — but when it gets cloudy, it falls to 10% when people in white clothes walk past a white fence, perhaps lowering overall accuracy over time to a very risky 80%.
For critical safety-related applications like this, it’s vital to ensure the model is trained by capturing enough images of people in different lighting conditions, and with sufficient diversity of garment types, fabric colours — plus skin tone, body size, age and gender. To do that, users should start with a moderate number of real images and add in many synthetic ones until the model discriminates to the specification.
But in less demanding, non-safety-related applications, perhaps discriminating between, say, limes and apples at a supermarket checkout, where a mistake would only cost a few pence, then synthetic data alone will give close to 100% accuracy and significant real data would simply not be needed. It’s possible with these sorts of applications, synthetic data can do much of the heavy lifting when it comes to training.
While it is of course up to the machine vision developer to decide how good is good enough, we’d suggest that around 90% synthetic data to 10% real is a typical ratio that can achieve desired results; there is both research validating this and empirical evidence this ratio has performed well in a great many use cases. But while Mindtech’s data generation platform, Chameleon, lets people create a great deal of synthetic data quickly, that choice must always be led by the needs of the problem visual AI developers are trying to solve.
Sometimes, however, it’s obvious where synthetic data is the way to go. Attempting to acquire enough real world images of people getting too close to a section of railway line, in my example above, would be prohibitive, for instance. You’d need to find either a disused line, which might not look like your piece of track, or pay to have a section of railway closed down for a time, and pay actors to get too close to the line. And that is both difficult and expensive, not to mention potentially hazardous.
And even then there’s a risk you won’t actually capture all the data you need: missing people wearing certain garments in troublesome colours, and in the right age groups or body shapes, perhaps — so you would need to go back, at great expense, to try and recapture more accurate data. So such scenarios are clear, compelling candidates for a synthetic data approach.
Using Synthetic Data to capture corner case data
And getting rid of that need for an extensive, and expensive, real world data gathering operation seriously reduces the training and testing time for the networks, getting them to market massively faster, too.
Despite that, there is no chance that visual AI systems designed to be deployed in the real world could ever entirely dispense with real data — as the network test data is always going to have to be authentic, so having at least some real world data in your training mix is always going to be a must. And thanks to our strategic partnership with world-leading AI data annotation company Appen, AI developers can now access the best of both worlds — automated synthetic and real-world data — at the same time, too.
That said, the speedy production of the synthetic data component in the mix — which is perfectly annotated by default, remember — will change the way AI development teams work. Using the platform, AI teams can create their own IP value by quickly making their own virtual worlds on Chameleon or use one of our pre-built ‘off-the-shelf’ training packs to recreate common scenes in markets such as retail and smart home. Fundamentally, the time Chameleon allows AI engineers and developers to save on cleaning, de-duping and labelling real world data enables more time to be spent on algorithm development.
Mindtech Chameleon “Training-Ready” Data Pack — station occupancy and safety monitoring
AI teams get a further productivity boost from Chameleon’s Curation Manager, too. After it has imported both the synthetic and real datasets, Curation Manager also allows the merged dataset to be queried in some powerful ways. For instance, if your application monitors social distancing in a mall, or perhaps ensures employees keep their distance from dangerous industrial robots, Curation Manager lets you query how many people are pictured in each training image, and what the spacing is in between them or between them and the robots. This way, data scientists can check that their mixed synthetic and real world data covers all the situations their visual AI model demands.
So the choice for AI teams today isn’t whether to work with synthetic data or real-world data. Successful teams know that the best results come from applying the best combination of both.
What’s the ideal mix of Synthetic and Real Data to boost AI Accuracy? was originally published in MindtechGlobal on Medium, where people are continuing the conversation by highlighting and responding to this story.