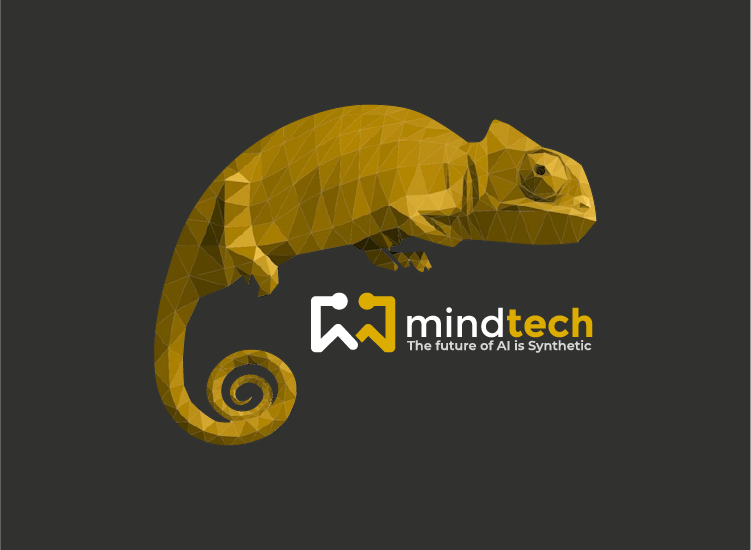
Share article:
Tags:
Why AI doesn’t see the world like us — and what that means for training data
By Peter McGuinness, VP Engineering, Mindtech
Synthetic images have become a vital part of the solution in the computer and machine vision sectors, allowing developers to train their visual AI models rapidly while also providing profound advantages — like automatic object annotation and complete privacy compliance, not to mention the ability to create any scene imaginable.
And yet, despite these very clear benefits, some AI engineers are still holding out against the idea of training models with synthetic images, preferring instead to only focus on real world imagery.
The reason for this, they argue, is that computer-generated synthetic images are not 100% photorealistic, so their models would be being trained using images that don’t accurately represent the real world — therefore rendering the training inaccurate.
Computers “see” images as a series of RGB values
This argument is not entirely founded, and misses a key benefit of synthetic data. The reason? That “real” data, in fact, only represents a snapshot taken with specific conditions, on a specific capture system, and may not be more representative of the scene to a target AI model than a synthetic image.
To use human preferences for aesthetically pleasing “real-world” images onto AI systems is unhelpful, and will not assist in the training of the AI system. AI models do not “see” like humans do and to an AI, the data is just that. This data must be helpful for the AI system to understand the structure and statistics of the problem in hand.
The reason? The content of a camera image depends on an extended pipeline of pixel manipulations, beginning at the lens and moving onto sensing, color filtering, spatial filtering, motion compensation, error correction and color correction. After that it is (usually lossily) compressed into a format like H.264/JPEG and presented as a final image.
The same Image processed via different pipelines — Humans likely prefer the left side of the image, but it is quite possible that your vision system is being delivered something closer to that on the right
It is important that in training an AI system, we provide matched data; data so the ML network can understand these characteristics of the capture system that will ultimately be used to provide the input to the AI when deployed. And therefore the data that will be inferenced, and ultimately provide the actionable output.
Mindtech’s Chameleon synthetic imaging engine can match the precise characteristics of the target deployment capture system. Images are output in both an ‘ideal’ form, without lens or imaging pipeline distortions; so can be easily retargeted at a future date to alternate target systems. In addition to this “golden” image, Chameleon will also transform the synthetic image using a plug-in model of the specific camera system, to provide matched data.
Setting cameras in Chameleon to match target domain requirements
So this sometime perceived “reality gap” from “real” images is in fact not justified. And we at Mindtech have demonstrated this in the real world, by training networks with synthetic data and tuning them until they perform extremely well; We have done this across multiple application domains, for many network types such as classification, detection, segmentation, pose and activity recognition. When combined with real data we have shown it will produce results better than any solutions based on real data alone.
This is not to say that we do not need to ensure there is a minimal gap in terms of “photo-realism” between synthetic and real-world data. We of course use state of the art graphic and animation techniques, as well as AI assistance such as GANs to minimize any gap. However, photorealism is just one aspect that we need to take care of; we need to address any gap in training data, for example this could be due to lack of data matching the deployment system characteristics, the deployment physical location, weather conditions, corner case and so on.
Ultimately this means training a model with the correct images, and providing the required coverage. Mindtech has now generated synthetic images to help many companies bridge data gaps in visual AI models that were malfunctioning. For instance, in one application, a system a company had developed to sense when people were present in a hazardous construction environment wasn’t working with sufficient accuracy with both missed and false positive detections.
Mindtech’s Chameleon: Curated training data, correctly oriented to target domain
On working with the team, we discovered that their model had been trained on people wearing ordinary work clothes — rather than the hi-viz jackets and hard hats that many ore in the target environment. By synthetically creating images of people in correct PPE gear using the Mindtech platform, the customer’s team were able to quickly close the gap — and the model was able to identify people correctly. It was equally important to model the cameras correctly as the low data rates were causing blocking artefacts.
Mindtech Chameleon simulates the compression artefacts; vital for accurate training
And in another data gap example, a system designed to sense empty car parking spots was not doing so. The training data had included thousands of pictures of cars but only ones shot at road level, whereas the car park cameras were look-down ones, high on poles, and so the system needed to know what cars look like from above. Again, closing the gap by training it on synthetic cars seen from overhead, the system was able to understand the correct perspective.
Chameleon: Using Synthetic Data to provide the required viewpoint
Time and again, we find that the data gap is much more significant than any perceived “photorealism gap”. What we’re finding working with customers is that in most cases what ultimately determines the accuracy and success of the AI is whether the training data is inadequate.
Chameleon does produce “Photoreal” images, but matching other elements of the image pipeline is of equal importance for training AI systems
Here at Mindtech we are constantly evolving our solution, narrowing any “gaps” in our customers training dataset, be they lack of specific corner case samples, lack of specific camera models or so on. We of course provide images that are “machine photorealistic” i.e. generated to be of use in training a machine to see.
Why AI doesn’t see the world like us — and what that means for training data was originally published in MindtechGlobal on Medium, where people are continuing the conversation by highlighting and responding to this story.