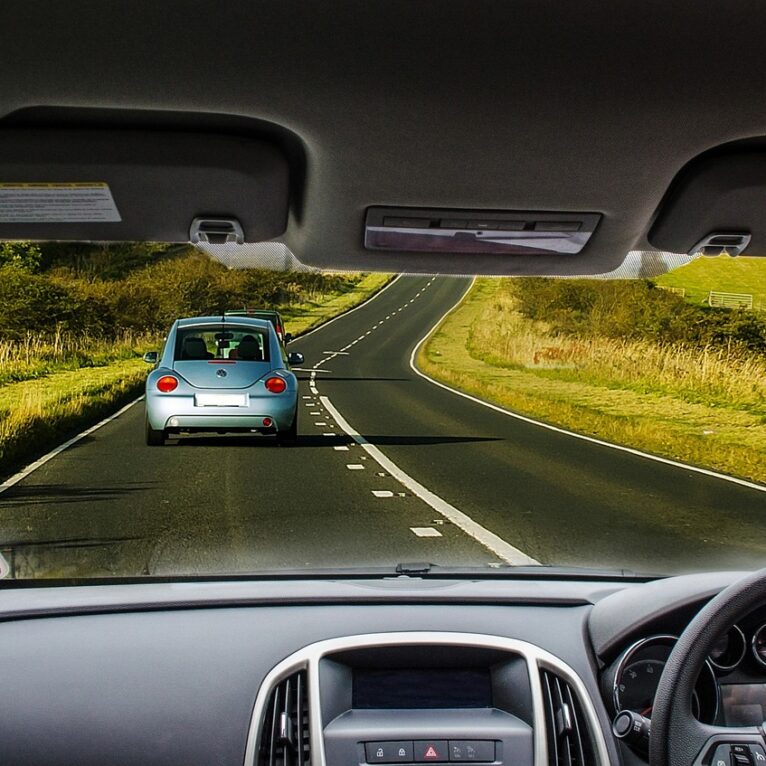
Share article:
Tags:
With a need to test the safety of vehicles in a myriad of ways, it makes sense that the automotive industry was one of the first proponents of synthetic data. The approach of using actors to recreate real-world and in-cabin experiences has transitioned into a virtual world that can provide safer and more efficient processes for testing driver safety, car navigation and security.
This rise in synthetic data can be attributed to the complex and challenging aspects of data collection in edge cases especially. As a result, a steady increase in its adoption is further anticipated over the coming years. In fact, according to a widely cited Gartner study, synthetic data across the training data market will account for 60 percent of all training data and overtake real world datasets by 2024. For the automotive industry, its benefits may make this transition even quicker.
Reflecting the real-world with diverse scenarios
While traditional use of synthetic data in the automotive industry has been used to test car safety and potential hazards, new applications such Mindtech’s Chameleon platform are enabling users to get a unique perspective on the visualisation of their environment (previously unavailable to them) through the use of multi-camera technology. These visual synthetic models can recreate and imagine real-world situations to help understand driver behaviour.
Automotive engineers are using the qualities of synthetic data to form virtual environments that simulate real-world driving conditions. This allows them to test many various and diverse scenarios without physical prototypes or expensive real-world tests, including driver in-cabin distractions — such as phone notifications and fatigue — passenger interactions, different weather conditions, traffic situations and road configurations.
For the models to perform accurately, a vast amount of data is needed. Clearly, trying to use real-world actors and cameras to act out every scenario would be incredibly time-consuming and costly. With synthetic data, it’s possible to alter any variable, such as any driver distractions, the number of passengers and their ages, to create a multitude of scenarios. This could even include rare edge case ones — such as freak weather events — that would be unfeasible to reenact in real life. Crucially, this method also bypasses any data privacy infringements.
Creating these diverse scenarios also helps to aid anomaly detection and fault diagnosis. By generating diverse synthetic data representing various fault conditions, for example, engineers can train algorithms to identify and diagnose anomalies and faults in the vehicle’s systems. This improves overall safety and reliability.
Synthetic data is increasingly being used to enhance various aspects of vehicle safety and protection. By leveraging synthetic data, manufacturers can improve the development, testing, and evaluation of security measures. Extensive datasets can be generated to account for various factors related to the driving environment, such as traffic volume and obstructions. It contributes to the continuous improvement of in-cabin security systems, protecting vehicles and their occupants from potential vulnerabilities.
Development challenges
The process of generating synthetic data for automotive development comes with its challenges. Its aim is to convey realism for reliable testing and calibration, but accurately reflecting real-world driving conditions, including variations in lighting, weather and object interactions, remains a challenge.
This task is heightened when it comes to rare edge cases and adequately representing infrequent and exceptional driving situations. These variabilities mean engineers need to implement thorough validation testing methods to ensure models trained on synthetic data perform well in real-world scenarios.
Moreover, automotive engineers have the juggling act of generating high-quality, large-scale synthetic datasets that mimic real-world scenarios while also maintaining data diversity, realism and adhering to privacy regulations. Organisations have to establish data usage policies that comply with laws and are conducive to fostering an ethical practice. This can all affect cost and time efficiency, requiring careful cost-benefit considerations.
Activating adoption and instilling trust
The availability of resources and type of use case can dictate the ease or difficulty of adopting synthetic data. For successful adoption, it’s imperative that automotive companies have access to skilled professionals, who understand synthetic data intricacies, and adequate computational resources, including high-performance computing and storage capabilities, for generating and processing synthetic data at scale.
Carrying out robust performance assessments can also bring to light the best ways to integrate synthetic data into existing workflows and systems. For successful integration, it’s paramount to assess the effectiveness of synthetic data models by setting benchmarks with real-world data comparisons.
The automotive industry is perhaps one of the most primed for using synthetic data. How quickly the industry enables its widespread adoption rests on its ability to overcome the above challenges, implement the necessary technology and resources, and instil a trust in its safety and reliability.
These data models have vast potential to grow further and become impressively sophisticated, helping to accurately reflect real-world driving conditions in countless ways and, ultimately, transform in-cabin safety.
Why synthetic data is the future fuel for in-cabin safety was originally published in MindtechGlobal on Medium, where people are continuing the conversation by highlighting and responding to this story.